Quant strategy overfitting intro
In trading, overfitting means that your model or backtest will seem to perform great on historical data, or backtest, but perform poorly on live trading data. This can make you implement a poor trading strategy or allocate more capital to a model than you should. Both are costly mistakes.
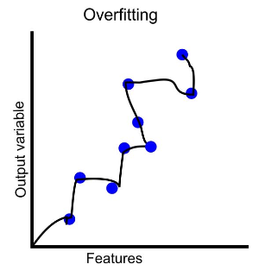
Does selling backtests work?
Sometimes we are approached by quants with a great backtest. They either want to sell their strategy or ask us to implement it and give them a share of the profits. (How) does this model work?
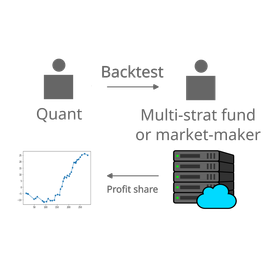
Tips and tricks to avoid overfitting in trading
Overfitting can cost you a lot of time and money. Here are a few trading-related tricks to avoid overfitting that are not known outside the algo-trading community.
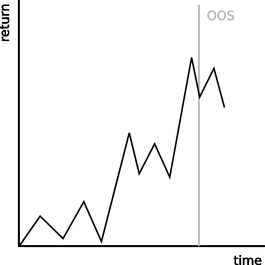
Building HFT algos with hftbacktest and Lake
Come back later for: Backtest high-sharpe HFT strategies with hftbacktest integrated with Lake
New posts are announced on RSS, twitter @jan_skoda or @crypto_lake_com, so follow us!